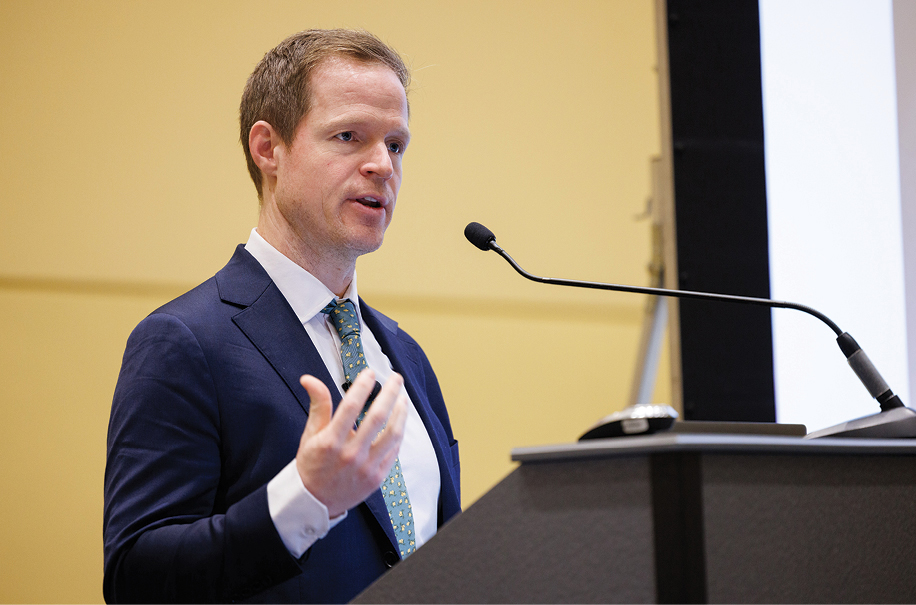
The AAOS Resident Assembly (RA) Education Forum, titled “AI in Orthopaedics: How We Got Here and Where We Are Going,” was held during the AAOS 2024 Annual Meeting in San Francisco and featured presentations from Kyle Kunze, MD; Justin Krogue, MD; and Michael Rivlin, MD, on foundations, current practices, and future directions of artificial intelligence (AI) in orthopaedics.
Fundamentals of using AI
Dr. Kunze, orthopaedic surgery resident at Hospital for Special Surgery, began with an overview of commonly used AI statistical methods, including information on how residents can utilize these tools themselves. “The time to understand AI is now, because it’s here to stay,” he said.
Dr. Kunze introduced several statistical approaches and methods of data interpretation using machine learning (ML). He noted that, although AI may be getting a lot of attention, at its core it is “not that different from traditional regression methods,” and many of the steps involved in data analysis using AI are the same as those required for traditional regression. He encouraged the continued use of traditional methods, which are often “just as powerful.” In some cases, the addition of AI does not improve data analysis. “AI’s not a magic solution or a panacea,” Dr. Kunze said. “In several studies, there’s no benefit compared to regression.” He also encouraged residents who want to use AI in statistical analyses to develop novel research questions that justify using this tool. “Avoid repackaging old data into studies with the buzzword ‘AI,’” he cautioned.
For developing and training models, Dr. Kunze recommended utilizing several different types of models at once to ensure that the selected model has the highest performance and most accuracy. He described his preferred model architectures, which include random forest, eXtreme gradient boosting, stochastic gradient boosting, support vector machine, neural network, and elastic-net penalized logistic regression. He also offered tips for assessing performance and fine tuning, validating, and deploying models. He closed with an overview of some of the tools on the horizon that could impact patient care, such as deep learning, which can be used to generate predictions based on image, video, and audio data, and natural language processing, which is utilized to develop large language models (e.g., ChatGPT) that can predict spoken or written language.
Applications of deep learning
Following Dr. Kunze was an informative discussion of the history and current applications of AI from Dr. Krogue, assistant professor of orthopaedic surgery at the University of California, San Francisco and clinical scientist in health AI at Google. Dr. Krogue outlined key differences between traditional statistical interference, which explains relationships between variables, and ML, which uses data to make predictions. He also differentiated “traditional” ML versus deep learning. While traditional ML utilizes “shallow” models to generate predictions in a few steps using algorithms such as logistics regression, deep learning utilizes neural networks, which are “deep” models with many intermediate steps of computation between inputs and outputs. Traditional ML models come with strong built-in (i.e., linear) assumptions about relationships between variables and outcomes, whereas deep learning has much weaker assumptions.
He demonstrated this difference with an example: assessing infection risk after knee arthroplasty, assuming that patients who are both smokers and either underweight or obese are at higher risk. The infection risk is non-linear because of the BMI range, so a traditional linear regression would fail to identify this relationship based on the data.
“Logistic regression assumes that there’s a linear relationship between your inputs and your outputs. Even if there’s a very real association, if it’s not linear, [a logistic regression model is] not going to find it,” Dr. Krogue said. In this example, a human would have to format the data in a way for the model to identify a linear relationship, such as separating BMI as “in range” and “out of range.” Deep learning, in contrast, would accurately predict infection risk because it can identify non-linear relationships.
Dr. Krogue discussed paradigm shifts in AI since the 1970s, highlighting what he called “narrow” AI that arose in the 2010s and generative AI tools of the 2020s. Narrow AI is adept at pattern recognition and trained to do specific tasks, such as labeling data. In orthopaedics, narrow AI’s applications are broad, including radiograph interpretation, task automation, and outcomes prediction. Generative AI is utilized to generate text, images, or other data and, importantly, can do tasks without specific training. Enabled by training on much larger datasets, generative AI models can generalize to new tasks when prompted. He offered an example from his own practice, where he utilized a large language model (GPT 3.5) to create a patient instruction write-up. In his prompt, he included the basic text from his patient treatment plan, specified the write-up should be at a sixth-grade reading level and expand any definitions, and added several examples. (To read more about generative AI in orthopaedics, see “Generative AI Could Transform Healthcare” in the March/April issue of AAOS Now.)
He noted some limitations of generative AI, specifically “hallucinations,” which are when the model makes up information to answer the prompt, such as creating a reference list with studies that are not from credible sources, not well done or significant, or just not real. These hallucinations may read well or seem legitimate, making them difficult to detect.
“You get this text that’s amazing, and it’s 99 percent right, but there’s some part that’s wrong, and it’s difficult to figure out what that might be,” he said. “Although I would also say that humans do this all the time, right?” Like human error, AI hallucinations are not a new problem, Dr. Kunze said, but they are an important problem for anyone using these tools.
Potential of AI in orthopaedic practice
Closing the session was Dr. Rivlin, hand and wrist surgeon with Rothman Orthopaedics, who discussed the use of AI in orthopaedic practice and addressed the hype and fears about the future of this technology. Many available AI tools can be utilized to reduce surgeons’ clerical burdens, but some of these tools are “not ready for primetime,” Dr. Rivlin said.
In a pilot study of an automated voice-transcription service, he found a significant amount of time was still spent reviewing the transcription and inputting data into the system. When extracting data from the transcription, the models struggled to pick up on nuances, such as information that was implied rather than directly stated.
In another pilot, Dr. Rivlin’s team compared a traditional call center to an AI-based, self-scheduled appointment system. With the AI-based system, patients answered a few questions, and the model would sort them according to type of physician and potential treatment (e.g., operative candidate, nonoperative treatment). Compared to the call center, Dr. Rivlin reported that the AI-based scheduling system resulted in a similar number of patients correctly identified as surgical candidates and for which kind of surgeon. At first, Dr. Rivlin was worried about implementing this system, thinking, “‘I’m going to see so much stuff that does not need hand surgery,’—I was wrong,” he said. “The surgical yield is the same, which is incredible,” he said. The tool was also successful at identifying the patient’s level of urgency and when the use of telehealth was appropriate. In the future, Dr. Rivlin believes that AI tools will reduce the workload and increase the accuracy of tasks like the check-in process, collection of medical history, patient counseling, and billing.
On the operative side, AI can help with preoperative planning, training, and even surgery itself using AI-powered surgical robots. Currently, these robots are aids for the surgeon, who leads and makes all surgical decisions. However, Dr. Rivlin assured, soon these robots will be able to work autonomously, including decision making. AI also has a potential role in designing custom implants. “We will be able to do truly patient-specific implants that are rapidly manufactured,” Dr. Rivlin predicted.
Looking ahead, Dr. Rivlin discussed smart implants, which can predict whether infection or wear are occurring and alert the physician directly. For example, “Mrs. Smith has an implant that is sending me a text [saying,] ‘There is something wrong around the implant, and I think I’m having an infection,’” Dr. Rivlin said. “There are companies that are currently working on it, and that is not science fiction anymore.”
Where can AI go wrong in the OR? Dr. Rivlin offered a few examples, such as a mixed-up radiograph interpretation, diagnosis based on false information or fiction, or recommendations of unconventional treatment options. A lot of the potential for error comes from issues with the data. “Garbage data in is garbage data out,” Dr. Rivlin said.
For now, with humans heavily regulating the use of these models, these issues are less of a threat. However, they may come to a head when AI tools are allowed to work autonomously in the clinic, either with decision making or directly during surgery. “The moment that AI will be actually touching the person or doing something physical is when things will get difficult,” Dr. Rivlin said. To the residents in the audience, he acknowledged, “Your generation will have to set boundaries for that.”
Rebecca Araujo is the managing editor of AAOS Now. She can be reached at raraujo@aaos.org.